Content Attributes
Finance is always about data, and this field has been using data science before the term or modern computers that help analyze it was a thing. The industry uses data in many ways and for different applications, all slightly different but interlinked. Let’s look at where experts are using it in finance.
Risk Analytics
Risk analytics helps companies increase trustworthiness among shareholders, make strategic decisions, and ensure their security. It measures the frequency and magnitude of loss, comparing both against how much benefit a business gains or how much damage it might have to deal with. Data informs all this, making stakeholders more aware of where they stand when making certain decisions and what impact these may have.
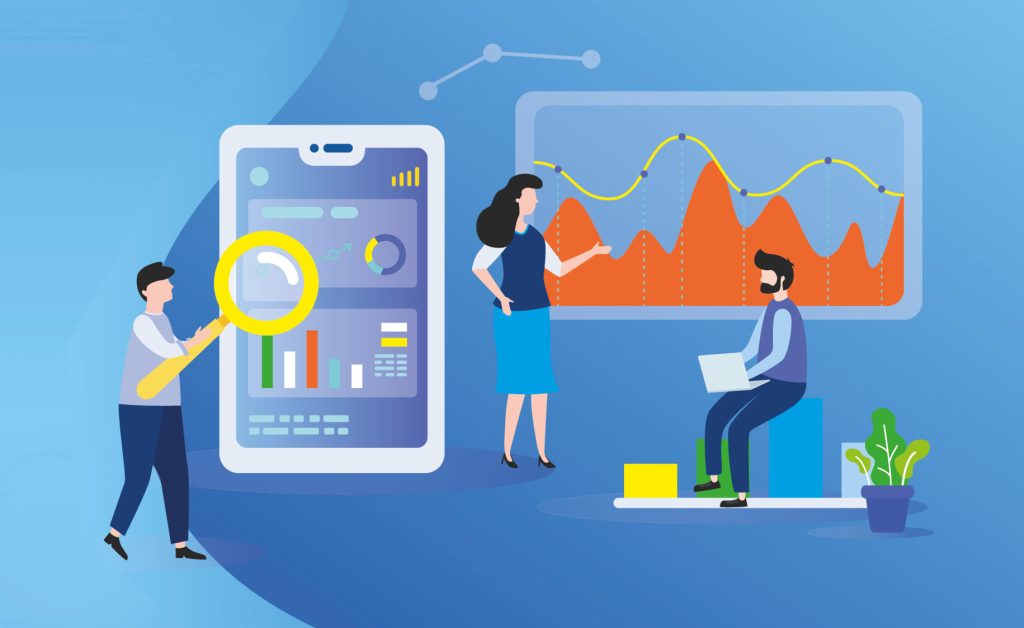
Risk management is a multidisciplinary field, requiring an intimate understanding of problem-solving, statistics, mathematics, and finance.
Data analytics is crucial for risk analysis because the amount of data businesses collect today is much more than they did in the past, and this data is more likely to be unstructured. Analytics helps clean and organize it, so it is useful to the company and its stakeholders.
Econometrics
Econometrics uses mathematical and statistical models to develop theories or test economic hypotheses. It also uses historical data to make predictions. Once it has subjected the data collected for different statistical trials, experts compare the results from this exercise against the theories they are testing to see how they hold up.
Econometrics is becoming a crucial part of the world as more businesses and organizations understand the value of using data to test hypotheses and economic models. This means holders of degrees in econometrics are in high demand and will continue to be in the future. This branch of finance and applied mathematics is already helping economies solve complex problems, further explaining why it is so important.
Real-time Analytics
Businesses used to analyze data in batches in the past. Because this data was historical and could not be collected and processed in real time, they did not have real-time insights into what was happening in the world and the markets.
However, technological advancements and dynamic data pipelines ensure minimal latency when accessing data. Businesses can collect as much data as they like and use analytics to understand it. This has enabled the use of data like credit scores and real-time purchases when making sales and marketing decisions and implementing strategies thereof.
Customer Data Management
Financial institutions and businesses need data about their customers for business intelligence applications. Businesses could use traditional data analytics tools to leverage this data, but now use powerful computers, machine learning, text analytics, data mining, and other technologies to generate insights from this data.
Additionally, they are using machine learning algorithms to analyze customer data to better understand financial trends and changes so they can react to and leverage what they learn.
Data analytics is massive and is being used in almost all industries as businesses look to make the most of the data they collect. Risk analytics, customer data analysis, econometrics, and real-time analytics depend on the capabilities made possible by this technological advancement.